
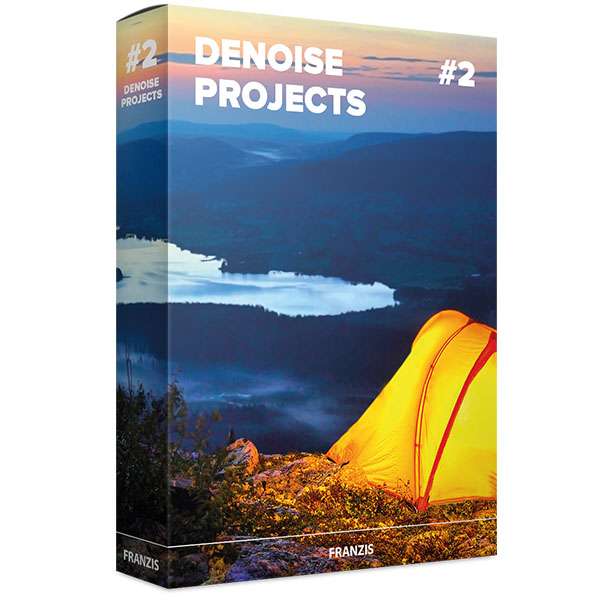
Thresholding is clearly not useful for denoising, but it is here to show that The result of Least Angle Regression is much more strongly biased: theĭifference is reminiscent of the local intensity value of the original image. Non-zero coefficients is a bit less biased than when keeping only one It can be seen from the plots that the results of Orthogonal Matching Pursuit (OMP) with two Reconstruction is perfect this will look like Gaussian noise. Noiseless) image, but here we start from the assumption that it is notĪ common practice for evaluating the results of image denoising is by lookingĪt the difference between the reconstruction and the original image. Performance could be achieved by fitting to an undistorted (i.e. Subsequently used to reconstruct the right half. The dictionary is fitted on the distorted left half of the image, and Of a raccoon face image using firstly online Dictionary Learning and
#Denoise photos online full#
(Noise is expected to be gaussian).To download the full example code or to run this example in your browser via Binder Image denoising using dictionary learning ¶Īn example comparing the effect of reconstructing noisy fragments cv.fastNlMeansDenoisingColored()Īs mentioned above it is used to remove noise from color images. Please visit first link in additional resources for more details on these parameters.

#Denoise photos online windows#
So we take a pixel, take small window around it, search for similar windows in the image, average all the windows and replace the pixel with the result we got.
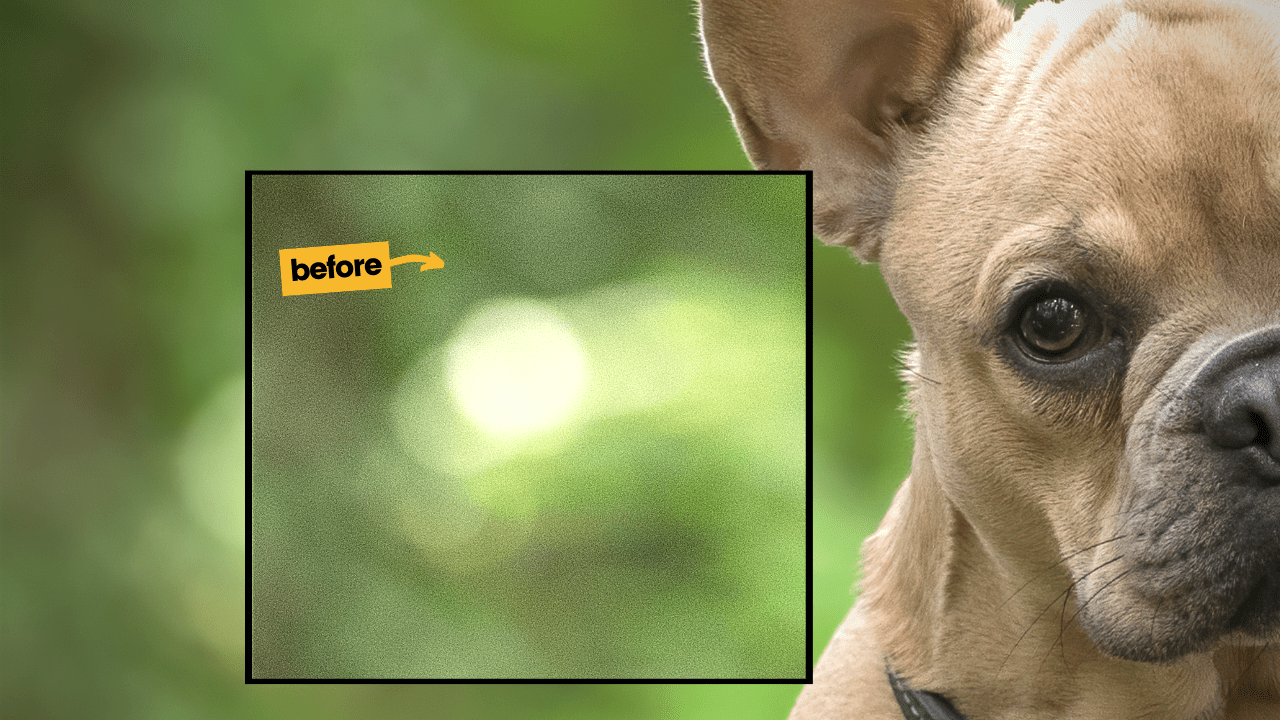
The blue patches in the image looks the similar. What about using these similar patches together and find their average? For that particular window, that is fine. Sometimes in a small neighbourhood around it.
#Denoise photos online Patch#
Chance is large that the same patch may be somewhere else in the image. Consider a small window (say 5x5 window) in the image. So idea is simple, we need a set of similar images to average out the noise.

Also often there is only one noisy image available. Unfortunately this simple method is not robust to camera and scene motions. Compare the final result and first frame. Then write a piece of code to find the average of all the frames in the video (This should be too simple for you now ). This will give you plenty of frames, or a lot of images of the same scene. Hold a static camera to a certain location for a couple of seconds. You can verify it yourself by a simple setup. Ideally, you should get \(p = p_0\) since mean of noise is zero. You can take large number of same pixels (say \(N\)) from different images and computes their average. Consider a noisy pixel, \(p = p_0 + n\) where \(p_0\) is the true value of pixel and \(n\) is the noise in that pixel. Noise is generally considered to be a random variable with zero mean. In short, noise removal at a pixel was local to its neighbourhood. In those techniques, we took a small neighbourhood around a pixel and did some operations like gaussian weighted average, median of the values etc to replace the central element. In earlier chapters, we have seen many image smoothing techniques like Gaussian Blurring, Median Blurring etc and they were good to some extent in removing small quantities of noise. You will see different functions like cv.fastNlMeansDenoising(), cv.fastNlMeansDenoisingColored() etc.You will learn about Non-local Means Denoising algorithm to remove noise in the image.
